diff options
Diffstat (limited to 'ml/README.md')
-rw-r--r-- | ml/README.md | 98 |
1 files changed, 28 insertions, 70 deletions
diff --git a/ml/README.md b/ml/README.md index f6fd923a..7f3ed276 100644 --- a/ml/README.md +++ b/ml/README.md @@ -1,14 +1,18 @@ -<!-- -title: Configure machine learning (ML) powered anomaly detection -custom_edit_url: https://github.com/netdata/netdata/edit/master/ml/README.md -description: This is an in-depth look at how Netdata uses ML to detect anomalies. -keywords: [machine learning, anomaly detection, Netdata ML] +<!-- +title: "Configure machine learning (ML) powered anomaly detection" +custom_edit_url: "https://github.com/netdata/netdata/edit/master/ml/README.md" +description: "This is an in-depth look at how Netdata uses ML to detect anomalies." +sidebar_label: "Configure machine learning (ML) powered anomaly detection" +learn_status: "Published" +learn_topic_type: "Tasks" +learn_rel_path: "Setup" --> + # Machine learning (ML) powered anomaly detection ## Overview -As of [`v1.32.0`](https://github.com/netdata/netdata/releases/tag/v1.32.0), Netdata comes with some ML powered [anomaly detection](https://en.wikipedia.org/wiki/Anomaly_detection) capabilities built into it and available to use out of the box, with zero configuration required (ML was enabled by default in `v1.35.0-29-nightly` in [this PR](https://github.com/netdata/netdata/pull/13158), previously it required a one line config change). +As of [`v1.32.0`](https://github.com/netdata/netdata/releases/tag/v1.32.0), Netdata comes with ML powered [anomaly detection](https://en.wikipedia.org/wiki/Anomaly_detection) capabilities built into it and available to use out of the box, with zero configuration required (ML was enabled by default in `v1.35.0-29-nightly` in [this PR](https://github.com/netdata/netdata/pull/13158), previously it required a one line config change). 🚧 **Note**: If you would like to get involved and help us with some feedback, email us at analytics-ml-team@netdata.cloud, comment on the [beta launch post](https://community.netdata.cloud/t/anomaly-advisor-beta-launch/2717) in the Netdata community, or come join us in the [🤖-ml-powered-monitoring](https://discord.gg/4eRSEUpJnc) channel of the Netdata discord. @@ -99,49 +103,7 @@ An ["anomaly detector"](#anomaly-detector) looks at all anomaly bits of a node. Essentially if the ["Node Anomaly Rate"](#node-anomaly-rate) (NAR) passes a defined threshold and stays above that threshold for a persistent amount of time, a "Node [Anomaly Event](#anomaly-event)" will be triggered. -These anomaly events are currently exposed via `/api/v1/anomaly_events` - -**Note**: Clicking the link below will likely return an empty list of `[]`. This is the response when no anomaly events exist in the specified range. The example response below is illustrative of what the response would be when one or more anomaly events exist within the range of `after` to `before`. - -https://london.my-netdata.io/api/v1/anomaly_events?after=1638365182000&before=1638365602000 - -If an event exists within the window, the result would be a list of start and end times. - -``` -[ - [ - 1638367788, - 1638367851 - ] -] -``` - -Information about each anomaly event can then be found at the `/api/v1/anomaly_event_info` endpoint (making sure to pass the `after` and `before` params): - -**Note**: If you click the below url you will get a `null` since no such anomaly event exists as the response is just an illustrative example taken from a node that did have such an anomaly event. - -https://london.my-netdata.io/api/v1/anomaly_event_info?after=1638367788&before=1638367851 - -``` -[ - [ - 0.66, - "netdata.response_time|max" - ], - [ - 0.63, - "netdata.response_time|average" - ], - [ - 0.54, - "netdata.requests|requests" - ], - ... -``` - -The query returns a list of dimension anomaly rates for all dimensions that were considered part of the detected anomaly event. - -**Note**: We plan to build additional anomaly detection and exploration features into both Netdata Agent and Netdata Cloud. The current endpoints are still under active development to power the upcoming features. +These anomaly events are currently exposed via the `new_anomaly_event` dimension on the `anomaly_detection.anomaly_detection` chart. ## Configuration @@ -152,7 +114,7 @@ To enable or disable anomaly detection: 2. In the `[ml]` section, set `enabled = yes` to enable or `enabled = no` to disable. 3. Restart netdata (typically `sudo systemctl restart netdata`). -**Note**: If you would like to learn more about configuring Netdata please see [the configuration guide](https://learn.netdata.cloud/guides/step-by-step/step-04). +**Note**: If you would like to learn more about configuring Netdata please see [the configuration guide](https://github.com/netdata/netdata/blob/master/docs/guides/step-by-step/step-04.md). Below is a list of all the available configuration params and their default values. @@ -162,6 +124,7 @@ Below is a list of all the available configuration params and their default valu # maximum num samples to train = 14400 # minimum num samples to train = 3600 # train every = 3600 + # number of models per dimension = 1 # dbengine anomaly rate every = 30 # num samples to diff = 1 # num samples to smooth = 3 @@ -169,12 +132,9 @@ Below is a list of all the available configuration params and their default valu # random sampling ratio = 0.2 # maximum number of k-means iterations = 1000 # dimension anomaly score threshold = 0.99 - # host anomaly rate threshold = 0.01000 - # minimum window size = 30.00000 - # maximum window size = 600.00000 - # idle window size = 30.00000 - # window minimum anomaly rate = 0.25000 - # anomaly event min dimension rate threshold = 0.05000 + # host anomaly rate threshold = 1.0 + # anomaly detection grouping method = average + # anomaly detection grouping duration = 300 # hosts to skip from training = !* # charts to skip from training = netdata.* ``` @@ -183,7 +143,7 @@ Below is a list of all the available configuration params and their default valu If you would like to run ML on a parent instead of at the edge, some configuration options are illustrated below. -This example assumes 3 child nodes [streaming](https://learn.netdata.cloud/docs/agent/streaming) to 1 parent node and illustrates the main ways you might want to configure running ML for the children on the parent, running ML on the children themselves, or even a mix of approaches. +This example assumes 3 child nodes [streaming](https://github.com/netdata/netdata/blob/master/streaming/README.md) to 1 parent node and illustrates the main ways you might want to configure running ML for the children on the parent, running ML on the children themselves, or even a mix of approaches. 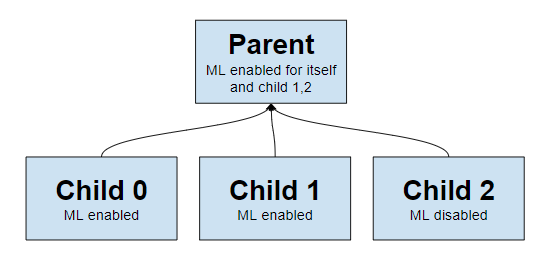 @@ -221,6 +181,7 @@ This example assumes 3 child nodes [streaming](https://learn.netdata.cloud/docs/ - `maximum num samples to train`: (`3600`/`86400`) This is the maximum amount of time you would like to train each model on. For example, the default of `14400` trains on the preceding 4 hours of data, assuming an `update every` of 1 second. - `minimum num samples to train`: (`900`/`21600`) This is the minimum amount of data required to be able to train a model. For example, the default of `900` implies that once at least 15 minutes of data is available for training, a model is trained, otherwise it is skipped and checked again at the next training run. - `train every`: (`1800`/`21600`) This is how often each model will be retrained. For example, the default of `3600` means that each model is retrained every hour. Note: The training of all models is spread out across the `train every` period for efficiency, so in reality, it means that each model will be trained in a staggered manner within each `train every` period. +- `number of models per dimension`: (`1`/`168`) This is the number of trained models that will be used for scoring. For example the default `number of models per dimension = 1` means that just the most recently trained model (covering up to the most recent `maximum num samples to train` of training data) for the dimension will be used to determine the corresponding anomaly bit. Alternatively, if you have `train every = 3600` and `number of models per dimension = 24` this means that netdata will store and use the last 24 trained models for each dimension when determining the anomaly bit, this means that for the latest feature vector in this configuration to be considered anomalous it would need to look anomalous across _all_ the models trained for that dimension in the last 24 hours. As such, increasing `number of models per dimension` may reduce some false positives since it will result in more models (covering a wider time frame of training) being used during scoring. - `dbengine anomaly rate every`: (`30`/`900`) This is how often netdata will aggregate all the anomaly bits into a single chart (`anomaly_detection.anomaly_rates`). The aggregation into a single chart allows enabling anomaly rate ranking over _all_ metrics with one API call as opposed to a call per chart. - `num samples to diff`: (`0`/`1`) This is a `0` or `1` to determine if you want the model to operate on differences of the raw data or just the raw data. For example, the default of `1` means that we take differences of the raw values. Using differences is more general and works on dimensions that might naturally tend to have some trends or cycles in them that is normal behavior to which we don't want to be too sensitive. - `num samples to smooth`: (`0`/`5`) This is a small integer that controls the amount of smoothing applied as part of the feature processing used by the model. For example, the default of `3` means that the rolling average of the last 3 values is used. Smoothing like this helps the model be a little more robust to spiky types of dimensions that naturally "jump" up or down as part of their normal behavior. @@ -228,40 +189,37 @@ This example assumes 3 child nodes [streaming](https://learn.netdata.cloud/docs/ - `random sampling ratio`: (`0.2`/`1.0`) This parameter determines how much of the available training data is randomly sampled when training a model. The default of `0.2` means that Netdata will train on a random 20% of training data. This parameter influences cost efficiency. At `0.2` the model is still reasonably trained while minimizing system overhead costs caused by the training. - `maximum number of k-means iterations`: This is a parameter that can be passed to the model to limit the number of iterations in training the k-means model. Vast majority of cases can ignore and leave as default. - `dimension anomaly score threshold`: (`0.01`/`5.00`) This is the threshold at which an individual dimension at a specific timestep is considered anomalous or not. For example, the default of `0.99` means that a dimension with an anomaly score of 99% or higher is flagged as anomalous. This is a normalized probability based on the training data, so the default of 99% means that anything that is as strange (based on distance measure) or more strange as the most strange 1% of data observed during training will be flagged as anomalous. If you wanted to make the anomaly detection on individual dimensions more sensitive you could try a value like `0.90` (90%) or to make it less sensitive you could try `1.5` (150%). -- `host anomaly rate threshold`: (`0.0`/`1.0`) This is the percentage of dimensions (based on all those enabled for anomaly detection) that need to be considered anomalous at specific timestep for the host itself to be considered anomalous. For example, the default value of `0.01` means that if more than 1% of dimensions are anomalous at the same time then the host itself is considered in an anomalous state. -- `minimum window size`: The Netdata "Anomaly Detector" logic works over a rolling window of data. This parameter defines the minimum length of window to consider. If over this window the host is in an anomalous state then an anomaly detection event will be triggered. For example, the default of `30` means that the detector will initially work over a rolling window of 30 seconds. Note: The length of this window will be dynamic once an anomaly event has been triggered such that it will expand as needed until either the max length of an anomaly event is hit or the host settles back into a normal state with sufficiently decreased host level anomaly states in the rolling window. Note: If you wanted to adjust the higher level anomaly detector behavior then this is one parameter you might adjust to see the impact of on anomaly detection events. -- `maximum window size`: This parameter defines the maximum length of window to consider. If an anomaly event reaches this size, it will be closed. This is to provide an upper bound on the length of an anomaly event and cost of the anomaly detector logic for that event. -- `window minimum anomaly rate`: (`0.0`/`1.0`) This parameter corresponds to a threshold on the percentage of time in the rolling window that the host was considered in an anomalous state. For example, the default of `0.25` means that if the host is in an anomalous state for 25% of more of the rolling window then and anomaly event will be triggered or extended if one is already active. Note: If you want to make the anomaly detector itself less sensitive, you can adjust this value to something like `0.75` which would mean the host needs to be much more consistently in an anomalous state to trigger an anomaly detection event. Likewise, a lower value like `0.1` would make the anomaly detector more sensitive. -- `anomaly event min dimension rate threshold`: (`0.0`/`1.0`) This is a parameter that helps filter out irrelevant dimensions from anomaly events. For example, the default of `0.05` means that only dimensions that were considered anomalous for at least 5% of the anomaly event itself will be included in that anomaly event. The idea here is to just include dimensions that were consistently anomalous as opposed to those that may have just randomly happened to be anomalous at the same time. +- `host anomaly rate threshold`: (`0.1`/`10.0`) This is the percentage of dimensions (based on all those enabled for anomaly detection) that need to be considered anomalous at specific timestep for the host itself to be considered anomalous. For example, the default value of `1.0` means that if more than 1% of dimensions are anomalous at the same time then the host itself is considered in an anomalous state. +- `anomaly detection grouping method`: The grouping method used when calculating node level anomaly rate. +- `anomaly detection grouping duration`: (`60`/`900`) The duration across which to calculate the node level anomaly rate, the default of `900` means that the node level anomaly rate is calculated across a rolling 5 minute window. - `hosts to skip from training`: This parameter allows you to turn off anomaly detection for any child hosts on a parent host by defining those you would like to skip from training here. For example, a value like `dev-*` skips all hosts on a parent that begin with the "dev-" prefix. The default value of `!*` means "don't skip any". -- `charts to skip from training`: This parameter allows you to exclude certain charts from anomaly detection. By default, only netdata related charts are excluded. This is to avoid the scenario where accessing the netdata dashboard could itself tigger some anomalies if you don't access them regularly. If you want to include charts that are excluded by default, add them in small groups and then measure any impact on performance before adding additional ones. Example: If you want to include system, apps, and user charts:`!system.* !apps.* !user.* *`. +- `charts to skip from training`: This parameter allows you to exclude certain charts from anomaly detection. By default, only netdata related charts are excluded. This is to avoid the scenario where accessing the netdata dashboard could itself trigger some anomalies if you don't access them regularly. If you want to include charts that are excluded by default, add them in small groups and then measure any impact on performance before adding additional ones. Example: If you want to include system, apps, and user charts:`!system.* !apps.* !user.* *`. ## Charts Once enabled, the "Anomaly Detection" menu and charts will be available on the dashboard. -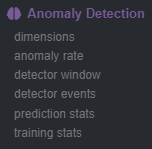 +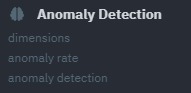 In terms of anomaly detection, the most interesting charts would be the `anomaly_detection.dimensions` and `anomaly_detection.anomaly_rate` ones, which hold the `anomalous` and `anomaly_rate` dimensions that show the overall number of dimensions considered anomalous at any time and the corresponding anomaly rate. - `anomaly_detection.dimensions`: Total count of dimensions considered anomalous or normal. - `anomaly_detection.dimensions`: Percentage of anomalous dimensions. -- `anomaly_detection.detector_window`: The length of the active window used by the detector. -- `anomaly_detection.detector_events`: Flags (0 or 1) to show when an anomaly event has been triggered by the detector. +- `anomaly_detection.anomaly_detection`: Flags (0 or 1) to show when an anomaly event has been triggered by the detector. Below is an example of how these charts may look in the presence of an anomaly event. Initially we see a jump in `anomalous` dimensions: -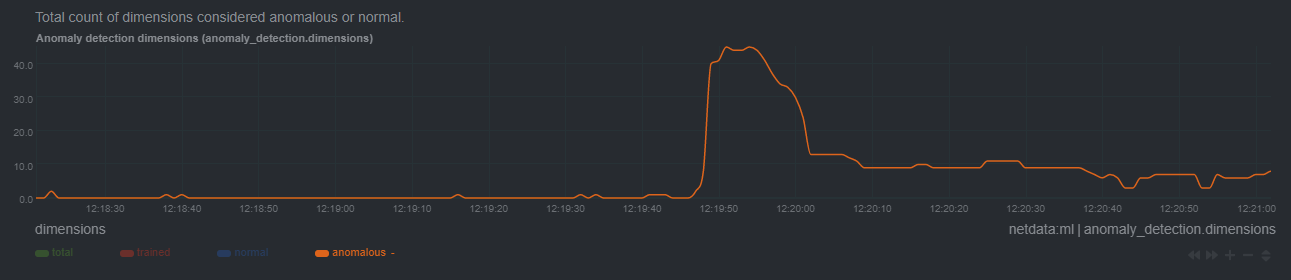 +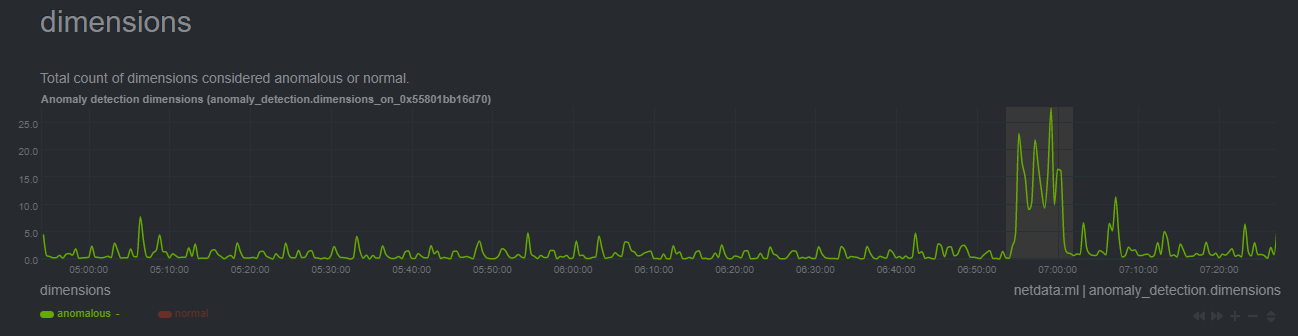 And a corresponding jump in the `anomaly_rate`: -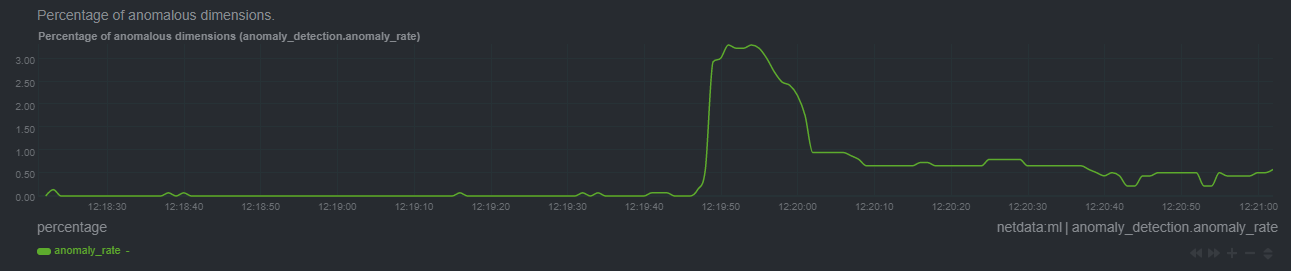 +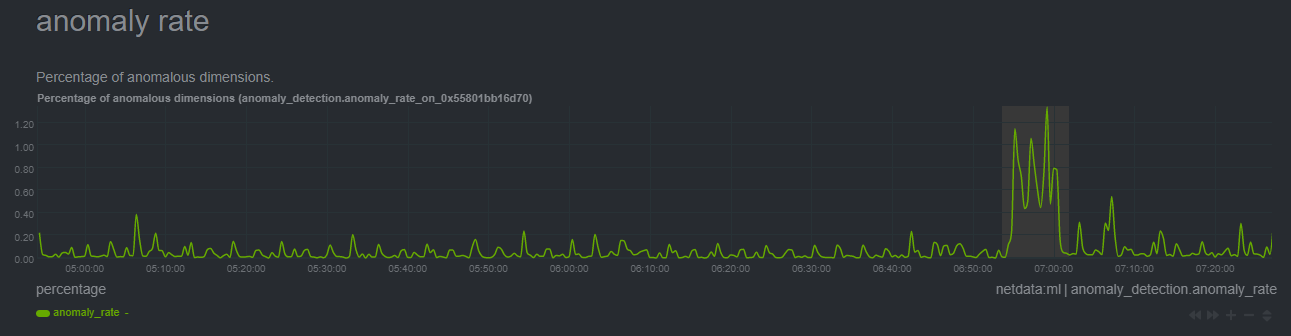 After a short while the rolling node anomaly rate goes `above_threshold`, and once it stays above threshold for long enough a `new_anomaly_event` is created: -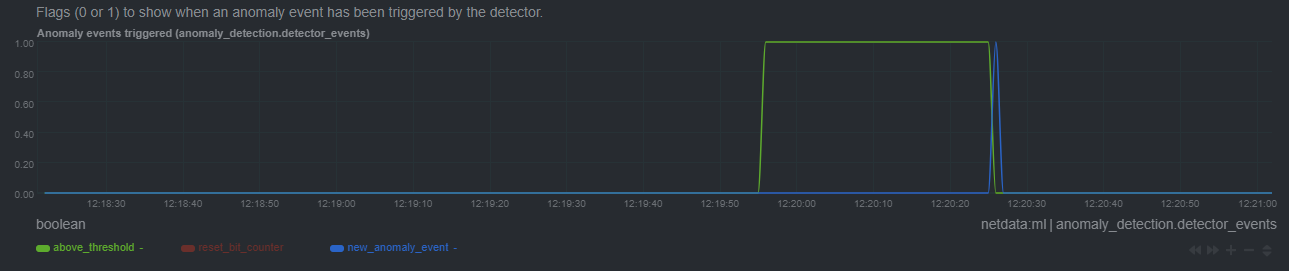 +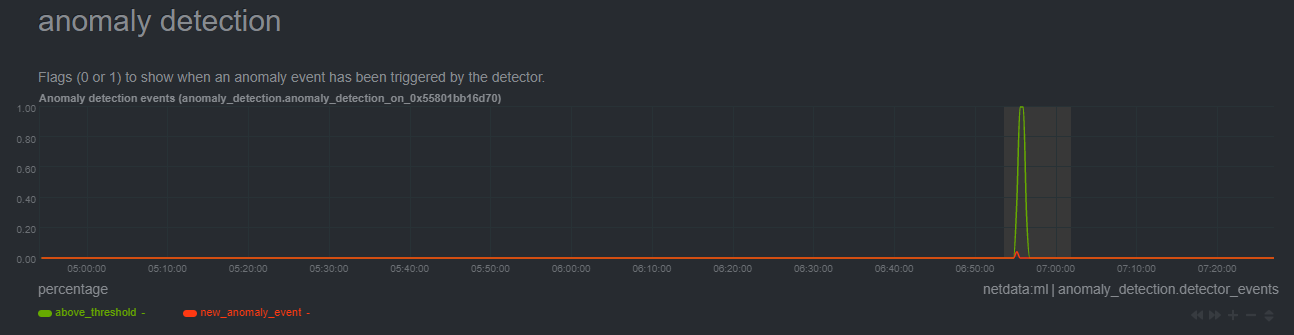 ## Glossary @@ -307,4 +265,4 @@ The anomaly rate across all dimensions of a node. - Netdata uses [dlib](https://github.com/davisking/dlib) under the hood for its core ML features. - You should benchmark Netdata resource usage before and after enabling ML. Typical overhead ranges from 1-2% additional CPU at most. - The "anomaly bit" has been implemented to be a building block to underpin many more ML based use cases that we plan to deliver soon. -- At its core Netdata uses an approach and problem formulation very similar to the Netdata python [anomalies collector](https://learn.netdata.cloud/docs/agent/collectors/python.d.plugin/anomalies), just implemented in a much much more efficient and scalable way in the agent in c++. So if you would like to learn more about the approach and are familiar with Python that is a useful resource to explore, as is the corresponding [deep dive tutorial](https://nbviewer.org/github/netdata/community/blob/main/netdata-agent-api/netdata-pandas/anomalies_collector_deepdive.ipynb) where the default model used is PCA instead of K-Means but the overall approach and formulation is similar. +- At its core Netdata uses an approach and problem formulation very similar to the Netdata python [anomalies collector](https://github.com/netdata/netdata/blob/master/collectors/python.d.plugin/anomalies/README.md), just implemented in a much much more efficient and scalable way in the agent in c++. So if you would like to learn more about the approach and are familiar with Python that is a useful resource to explore, as is the corresponding [deep dive tutorial](https://nbviewer.org/github/netdata/community/blob/main/netdata-agent-api/netdata-pandas/anomalies_collector_deepdive.ipynb) where the default model used is PCA instead of K-Means but the overall approach and formulation is similar. |